
A.I. has a discrimination problem. In banking, the consequences can be severe
More Videos
Published
2 years agoon
By
adminArtificial intelligence algorithms are increasingly being used in financial services — but they come with some serious risks around discrimination.
Sadik Demiroz | Photodisc | Getty Images
AMSTERDAM — Artificial intelligence has a racial bias problem.
From biometric identification systems that disproportionately misidentify the faces of Black people and minorities, to applications of voice recognition software that fail to distinguish voices with distinct regional accents, AI has a lot to work on when it comes to discrimination.
And the problem of amplifying existing biases can be even more severe when it comes to banking and financial services.
Deloitte notes that AI systems are ultimately only as good as the data they’re given: Incomplete or unrepresentative datasets could limit AI’s objectivity, while biases in development teams that train such systems could perpetuate that cycle of bias.
A.I. can be dumb
Nabil Manji, head of crypto and Web3 at Worldpay by FIS, said a key thing to understand about AI products is that the strength of the technology depends a lot on the source material used to train it.
“The thing about how good an AI product is, there’s kind of two variables,” Manji told CNBC in an interview. “One is the data it has access to, and second is how good the large language model is. That’s why the data side, you see companies like Reddit and others, they’ve come out publicly and said we’re not going to allow companies to scrape our data, you’re going to have to pay us for that.”
As for financial services, Manji said a lot of the backend data systems are fragmented in different languages and formats.
“None of it is consolidated or harmonized,” he added. “That is going to cause AI-driven products to be a lot less effective in financial services than it might be in other verticals or other companies where they have uniformity and more modern systems or access to data.”

Manji suggested that blockchain, or distributed ledger technology, could serve as a way to get a clearer view of the disparate data tucked away in the cluttered systems of traditional banks.
However, he added that banks — being the heavily regulated, slow-moving institutions that they are — are unlikely to move with the same speed as their more nimble tech counterparts in adopting new AI tools.
“You’ve got Microsoft and Google, who like over the last decade or two have been seen as driving innovation. They can’t keep up with that speed. And then you think about financial services. Banks are not known for being fast,” Manji said.
Banking’s A.I. problem
Rumman Chowdhury, Twitter’s former head of machine learning ethics, transparency and accountability, said that lending is a prime example of how an AI system’s bias against marginalized communities can rear its head.
“Algorithmic discrimination is actually very tangible in lending,” Chowdhury said on a panel at Money20/20 in Amsterdam. “Chicago had a history of literally denying those [loans] to primarily Black neighborhoods.”
In the 1930s, Chicago was known for the discriminatory practice of “redlining,” in which the creditworthiness of properties was heavily determined by the racial demographics of a given neighborhood.
“There would be a giant map on the wall of all the districts in Chicago, and they would draw red lines through all of the districts that were primarily African American, and not give them loans,” she added.
“Fast forward a few decades later, and you are developing algorithms to determine the riskiness of different districts and individuals. And while you may not include the data point of someone’s race, it is implicitly picked up.”
Indeed, Angle Bush, founder of Black Women in Artificial Intelligence, an organization aiming to empower Black women in the AI sector, tells CNBC that when AI systems are specifically used for loan approval decisions, she has found that there is a risk of replicating existing biases present in historical data used to train the algorithms.
“This can result in automatic loan denials for individuals from marginalized communities, reinforcing racial or gender disparities,” Bush added.
“It is crucial for banks to acknowledge that implementing AI as a solution may inadvertently perpetuate discrimination,” she said.
Frost Li, a developer who has been working in AI and machine learning for over a decade, told CNBC that the “personalization” dimension of AI integration can also be problematic.
“What’s interesting in AI is how we select the ‘core features’ for training,” said Li, who founded and runs Loup, a company that helps online retailers integrate AI into their platforms. “Sometimes, we select features unrelated to the results we want to predict.”
When AI is applied to banking, Li says, it’s harder to identify the “culprit” in biases when everything is convoluted in the calculation.
“A good example is how many fintech startups are especially for foreigners, because a Tokyo University graduate won’t be able to get any credit cards even if he works at Google; yet a person can easily get one from community college credit union because bankers know the local schools better,” Li added.
Generative AI is not usually used for creating credit scores or in the risk-scoring of consumers.
“That is not what the tool was built for,” said Niklas Guske, chief operating officer at Taktile, a startup that helps fintechs automate decision-making.
Instead, Guske said the most powerful applications are in pre-processing unstructured data such as text files — like classifying transactions.
“Those signals can then be fed into a more traditional underwriting model,” said Guske. “Therefore, Generative AI will improve the underlying data quality for such decisions rather than replace common scoring processes.”
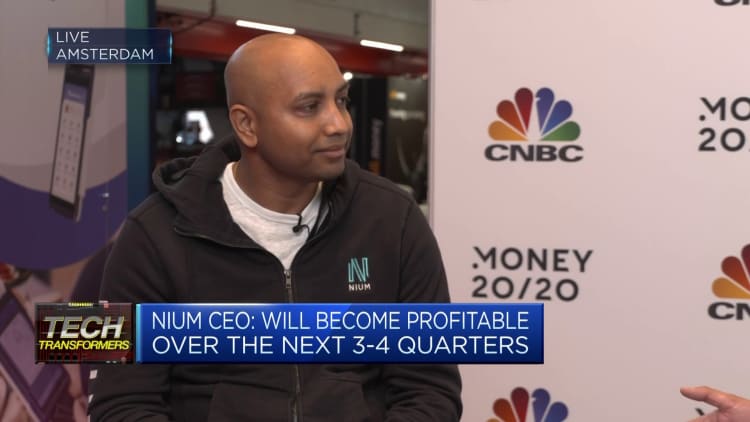
But it’s also difficult to prove. Apple and Goldman Sachs, for example, were accused of giving women lower limits for the Apple Card. But these claims were dismissed by the New York Department of Financial Services after the regulator found no evidence of discrimination based on sex.
The problem, according to Kim Smouter, director of anti-racism group European Network Against Racism, is that it can be challenging to substantiate whether AI-based discrimination has actually taken place.
“One of the difficulties in the mass deployment of AI,” he said, “is the opacity in how these decisions come about and what redress mechanisms exist were a racialized individual to even notice that there is discrimination.”
“Individuals have little knowledge of how AI systems work and that their individual case may, in fact, be the tip of a systems-wide iceberg. Accordingly, it’s also difficult to detect specific instances where things have gone wrong,” he added.
Smouter cited the example of the Dutch child welfare scandal, in which thousands of benefit claims were wrongfully accused of being fraudulent. The Dutch government was forced to resign after a 2020 report found that victims were “treated with an institutional bias.”
This, Smouter said, “demonstrates how quickly such disfunctions can spread and how difficult it is to prove them and get redress once they are discovered and in the meantime significant, often irreversible damage is done.”
Policing A.I.’s biases
Chowdhury says there is a need for a global regulatory body, like the United Nations, to address some of the risks surrounding AI.
Though AI has proven to be an innovative tool, some technologists and ethicists have expressed doubts about the technology’s moral and ethical soundness. Among the top worries industry insiders expressed are misinformation; racial and gender bias embedded in AI algorithms; and “hallucinations” generated by ChatGPT-like tools.
“I worry quite a bit that, due to generative AI, we are entering this post-truth world where nothing we see online is trustworthy — not any of the text, not any of the video, not any of the audio, but then how do we get our information? And how do we ensure that information has a high amount of integrity?” Chowdhury said.
Now is the time for meaningful regulation of AI to come into force — but knowing the amount of time it will take regulatory proposals like the European Union’s AI Act to take effect, some are concerned this won’t happen fast enough.
“We call upon more transparency and accountability of algorithms and how they operate and a layman’s declaration that allows individuals who are not AI experts to judge for themselves, proof of testing and publication of results, independent complaints process, periodic audits and reporting, involvement of racialized communities when tech is being designed and considered for deployment,” Smouter said.
The AI Act, the first regulatory framework of its kind, has incorporated a fundamental rights approach and concepts like redress, according to Smouter, adding that the regulation will be enforced in approximately two years.
“It would be great if this period can be shortened to make sure transparency and accountability are in the core of innovation,” he said.

You may like
Technology
Figma CEO’s path from college dropout and Thiel fellow to tech billionaire
Published
13 hours agoon
August 3, 2025By
admin
Dylan Field, co-founder and CEO of Figma, signs the guestbook on the floor of the New York Stock Exchange in New York on July 31, 2025.
Michael Nagle | Bloomberg | Getty Images
Mark Zuckerberg may be the most famous college-dropout-turned-tech-billionaire. Dylan Field is the latest, after his design startup Figma soared in its stock market debut this week.
The two entrepreneurs have something else in common: close ties to Peter Thiel.
Zuckerberg got his first outside check for Facebook from Thiel in 2004, soon before leaving Harvard University to build his social network in Silicon Valley. Facebook went public in 2012, the same year that Field scored a Thiel Fellowship, which gives money “to young people who want to build new things instead of sitting in a classroom.” Over 300 people have been selected since its inception in 2011.
Field, now 33, was part of the second batch of Thiel fellows, a group of 20 entrepreneurs who each took home $100,000. The program doubled that sum earlier this year. Like Zuckerberg, Field came to Thiel from the Ivy League, having spent two and a half years at Brown University in Providence, Rhode Island.
On Thursday, Figma’s stock price more than tripled in its first day of trading on the New York Stock Exchange. It rose again on Friday, wrapping up the week with a fully diluted market cap above $71 billion. Field’s stake is worth about $6.6 billion. Zuckerberg, meanwhile, is now the world’s third-richest person, with a net worth of over $260 billion.
While the contours of Field’s story may sound familiar, he’s a very different kind of character.
“Dylan is, by far, the most humble billionaire I’ve ever met,” said Joshua Browder, CEO of legal services startup DoNotPay and a former Thiel fellow.

Mike Gibson, who used to help run the fellows program as vice president for grants at the nonprofit Thiel Foundation, contrasts Field with another tech luminary.
“He’s kind of like the anti-Steve Jobs,” said Gibson, a co-founder of 1517 Fund, a venture firm that prides itself on investing in dropouts. “When it comes to Jobs’ legend as this hard-charging a–hole, Dylan is the opposite.”
The Apple co-founder, who dropped out of college after one semester, died of cancer in 2011, as his company was on its way to becoming the most valuable business in the world.
Field was poised to officially enter the billionaire ranks almost three years ago. With Figma having emerged as a leader in web-based tools for designing apps and websites, Adobe agreed to snap up its budding rival for $20 billion. But regulators in the U.K. said the tie-up would’ve hurt competition, and the companies scrapped the transaction in late 2023. Adobe payed Figma a $1 billion breakup fee.
Figma’s IPO this week represented not only a massive valuation markup for the company but also served as a banner event for Silicon Valley, which has seen a dearth of high-profile IPOs since the market cratered in early 2022 due to soaring inflation and rising interest rates.
“The most important thing to remind myself of, the team of, is share price is a moment in time,” Field told CNBC’s “Squawk Box” on Thursday. “We’re going to see all sorts of behavior probably today, over the weeks ahead.”
Figma declined to make Field available for an interview for this story.
Field’s trek back to the Bay Area, where he’d grown up, began with a TechCrunch article about the fellowship. He submitted his application two hours before the deadline, on New Year’s Eve of 2011, while he was a junior at Brown. He left out his SAT scores.

“It is my belief that the SAT is a poor reflection of aptitude and can easily be gamed,” he wrote in his application, which he posted on LinkedIn years later. In the essay section, he was asked to offer a highly controversial take.
“Chocolate is repulsive,” he wrote. “Even the smell of it makes me want to vomit.”
In response to a question about how he was going to change the world, Field said he was going to build better software for drones, and that he would “cofound a company with the smartest programmer I know and work on this problem.”
That co-founder was Evan Wallace, who had been a teaching assistant for some of Field’s courses at Brown. Wallace was technologically gifted, earning the nickname “computer Jesus,” or CJ. But he was already 20, meaning he was too old to be eligible for a Thiel Fellowship.
Field scored the $100,000 from Thiel, and shared it with Wallace, convincing him to leave his academic pursuits. The pair moved into a small apartment in Palo Alto, California.
The drone software plan had gone out the window. Wallace wanted to develop something related to WebGL, a graphics rendering system for web browsers. A year later, they were showing investors a slick browser-based demo that allowed for the movement of a ball in a pool of water.
‘Anyone can be creative’
The obvious competitive target was Adobe, which was ending development of Fireworks, an app design product that it acquired with the 2005 Macromedia purchase.
“We thought, ‘Wait, maybe there’s an opportunity here,'” Field said on a podcast earlier this year.
“What we’re trying to do is make it so that anyone can be creative, by creating free, simple creative tools in the browser,” Field said in a 2012 interview for a CNBC special on the Thiel Fellowship.
In 2013, the founders started talking with investors about raising a seed round. Field showed the pool water demo to John Lilly of Greylock Partners at a Starbucks in Palo Alto. Lilly had previously been CEO of Mozilla, where an engineer developed software that led to WebGL. He was impressed with what he was seeing, but he didn’t think it had much economic potential.
Figma took on seed funding from Index Ventures and other investors. The founders assembled a small group of employees at an office in Palo Alto. Progress was slow. Early versions of the product failed to impress potential users. Field was micromanaging.
When Figma would show the product to companies in the Bay Area, reception wasn’t always great. Stress was building. Lilly, who ended up leading Figma’s Series A round in 2014, came to the company’s San Francisco headquarters the following August as struggles were mounting. Employees wanted changes.
“We both heard it,” said Danny Rimer, the Index partner who led the seed funding, referring to conversations he and Lilly were having with staffers about Field.
“We sat down with him and explained to him the situation,” Rimer said. “We heard it and we sort of said, ‘Look, this is an impasse. You’re going to have to adapt and change.’ And he heard it and he changed. I think that’s such a great character trait of Dylan, is to hear the information, be objective about it, process it and accept it and act accordingly, if it makes sense.”
Dylan Field, co-founder and CEO of Figma, speaks at the startup’s Config conference in San Francisco on May 10, 2022.
Figma
Around that time, Sho Kuwamoto joined the company. Kuwamoto brought with him experience from Macromedia and Adobe. Four months later, Figma launched its debut product in a free preview.
Field got involved with users. He replied to people on social media who were posting about Figma, telling them they were receiving access to the preview. He also sought out prominent designers.
Companies like Coda and Uber became early adopters. Some designers were excited by the idea of sharing documents by copying and pasting a URL, instead of having to deal with versions, formats and updates. Figma operated in the cloud, providing all the necessary computing infrastructure, so users didn’t need their own powerful graphics cards.
It wasn’t until September 2016 that Figma made the design editor available for free to the general public and made it possible for multiple designers to make changes in a single file simultaneously. That became the killer feature.
The software started gaining traction inside Microsoft. But there was an issue. Microsoft feared that Figma’s lack of a clear business model might lead to a burial in the startup graveyard. Jon Friedman, a design executive at the software giant, visited Figma’s headquarters to deliver the message, Field told CNBC in 2022.
“Look, we’re all worried you’re going to die as a company,” Field recalled Friedman telling him.
The following year, Figma introduced its first paid tier.
By the time venture stalwart Sequoia Capital came on board in 2019, Figma was a hot commodity, raising its Series C round at a $440 million valuation. Sequoia partner Andrew Reed said some of his firm’s portfolio companies had started migrating to Figma, and founders were using it for pitch decks.
“Companies often will show prototypes in board meetings of new products they want to build, and so the first thing we saw a lot of Figma links for was that,” Reed said in an interview this week.
“It was a very easy investment,” Reed said. “We went through some of our old investment voting data. I think Figma might have been the highest vote we ever had for an investment.”
Sequoia’s extensive roster of winners over the decades includes Apple, Google, LinkedIn, Zoom and WhatsApp.
The Adobe period
Financial analysts covering Adobe started asking about Figma. Adobe, which had released the XD app for user experience design, responded, adding the startup to its official list of competitors.
But Adobe’s market capitalization sat above $170 billion, and Figma wasn’t even a “unicorn,” a status reserved for startups worth at least $1 billion. Field told Forbes that some job candidates were hesitant to join because of the modest valuation. In 2020, the company raised a funding round from Andreessen Horowitz at a $2 billion valuation.
Then came Covid. Offices closed. The world went remote overnight. Figma’s collaboration capability suddenly became critical to the way many more people worked.
“We asked ourselves: how can we help teams connect, have fun and enter a flow state during the earliest stages of the design process?” Field later wrote on Twitter.
The result was FigJam, a digital whiteboard that became Figma’s second product, and represented a key step toward diversification.
The Adobe noise continued to get louder. In 2020, Field had discussions with Adobe executive Scott Belsky about a partnership or acquisition, but Field chose to stay the course. Adobe CEO Shantanu Narayen talked to Field about a possible deal in early 2021, but again the Figma CEO demurred, opting to raise a round at a $10 billion valuation.
“Our goal is to be Figma not Adobe,” Field wrote in a 2021 tweet.
The environment quickly changed. By early 2022, with the Fed lifting interest rates to fight inflation, investors were selling out of high-growth tech and rotating into businesses with predictable profits. Sequoia was encouraging its startups to reduce costs.
David Wadhwani, president of Adobe’s Digital Media unit, speaks at Adobe’s MAX conference in Los Angeles, October 2022.
Adobe
Belsky again approached Field in April of that year, this time alongside David Wadhwani, who was leading Adobe’s digital media business.
“Mr. Field expressed openness to understanding the terms of a potential acquisition of Figma by Adobe, and Mr. Field, Mr. Belsky and Mr. Wadhwani continued their discussion of the potential benefits of a combination the following week,” Adobe stated in a regulatory filing.
Field was considering the implications of the rise of artificial intelligence.
“Look, when we did the deal with Adobe in the first place, my head space in 2022 was, “Oh my god, AI is coming. This is clearly exponential as a technology. I don’t know what this does to us. Is this one-tenth our market, is it 10x our market? What does it mean for creatives and designers?” Field said in an interview with The Verge last year. “And I was like, it’s better to team up in this world with Adobe and to navigate this together and to figure this out together than it is to go it alone.”
In September 2022, Adobe agreed to buy Figma for about $20 billion, announcing that Field would remain in charge of his part of the business and would report to Wadhwani.
“Adobe has a unique opportunity to usher in a world of collaborative creativity,” Narayen told analysts on a conference call the day of the agreement. “In my conversations with Dylan at Figma, it became abundantly clear that together we could accelerate this new vision, delivering great value to our customers and shareholders.”
That opportunity never came. An intensifying regulatory environment in the U.S. and Europe had made sizable tech deals more burdensome. Adobe was suddenly in the crosshairs, and the transaction was hitting repeated hurdles.
“We’re worried this deal could stifle innovation and lead to higher costs for companies that rely on Figma and Adobe’s digital tools — as they cease to compete to provide customers with new and better products,” Sorcha O’Carroll, an official at the U.K. Competition and Markets Authority, said in a press release in mid-2023.
Around that time, Field announced another step toward product diversification by introducing Dev Mode, which turns Figma designs into source code that can serve as a starting point for software developers. The reveal came at Figma’s Config user conference in San Francisco, which attracted 8,000 attendees.
The U.K.’s investigation dragged on for months. Field was pulling double duty running the company and engaging with regulators. Adobe had said it expected to complete the deal in 2023, but time was running out. Regulators were proposing remedies that the parties didn’t like.
“Even toward the final months, there were these moments of, ‘Oh, this is going to go through,’ and moments of, ‘F—, what are we doing?'” Field told The Verge. “And obviously at the end, there’s a mutual understanding of,’ This decision has been made for us and let’s call it.'”
On a Sunday in December 2023, Field gathered board members for a 10-minute call, informing them that the deal was off. The official statement followed early on Monday morning.
“It’s frustrating and sad that we’re not able to complete this,” Field told The New York Times.
Not everyone in Field’s orbit saw it that way. Grammarly CEO Shishir Mehrotra, a friend of Field’s and longtime Figma user, said the whole ordeal was having an impact.
“You could see it in his face,” Mehrotra said of Field, adding that he was relieved when he learned Figma would remain independent. “He was getting older right in front of us.”
But Figma had some business concerns. Its net dollar retention rate, a measurement of the company’s ability to sell more to existing customers, slid from 159% in the first quarter of 2023 to 122% by the end of the year, according to Figma’s IPO prospectus. Figma chalked it up to a tough comparison from the year before, thanks to the launch of FigJam, and economic uncertainty that caused some clients to reduce seat counts. The retention rate bounced back to 132% in the first quarter of 2025.
During the 2023 winter holidays, Field considered ways to rally the workforce. After the new year, he announced internally that Figma would give extra equity to employees who joined or received promotions following the acquisition announcement, because the valuation was going back down to $10 billion. He said any employees who wished to leave would get three months of severance, with no hard feelings.
Fewer than 5% of staffers took him up on the offer.
Pivot to prompting
As Figma pursues a go-it-alone strategy, it faces an existential question: Is the company ready for a future dominated by AI?
In May, Field took the stage at Figma’s user conference before 8,500 attendees at San Francisco’s Moscone Center, wearing a black “Config 2025” T-shirt. He walked the crowd through a slew of new products, including Figma Make, which draws on Claude 3.7 Sonnet, a large language model from AI startup Anthropic.
“With Figma Make, you could take an existing design and prompt your way to a fully coded prototype,” Field said.
A product manager, Holly Li, came up for a demo. At a laptop, she copied the design for a music player in the Figma editor and pasted it into a chat box, typing instructions to rotate the album art like a record while a song is playing. She showed apps created with Figma Make, eliciting some cheers, and returned to the demo.
“Okay. This time, the model had a little bit of difficulty, but that’s okay,” she said. The cloudy background image from the original design was gone, and track names became difficult to read. The crowd was silent. She brought up a working version in a different browser tab.
The feature went live last week. Mehrotra said it’s off to a good start.
Other products in the market were built with generative AI in mind. They include Lovable, Miro’s Uizard and Vercel’s v0. Brent Stewart, an analyst at Gartner, said that Figma is “utterly, utterly dominant” in design but that some of the offerings from other companies look more impressive.
Andrew Chan, a former Figma software engineer, wrote in a blog post last year that “an interesting and ongoing question is whether Figma can repeat the success it had in design with other products.”
Nadia Eldeib, a former Lyft product manager and CEO of startup CodeYam, tried Figma Make before the broad launch and put it up against Lovable and v0. Writing on Substack, she said it appeared to be at an earlier stage.
It’s the sort of feedback that Field will read and send to his employees, known as Figmates. He reads support tickets and mentions of Figma’s name on X, formerly Twitter. He took no time off to address such matters on the very day that his company was conducting its IPO, ultimately pricing shares $1 above the expected range.
Yianni Mathioudakis, a creative director in Maryland, tagged Figma in a post on Wednesday, asking if anyone had found a way to take a Figma Make design and bring it into the main design editor.
“Hi Yianni, we are working towards this and very excited about what it will unlock!” Field replied. “Please keep the Make feedback coming!”

Technology
Tesla must pay portion of $329 million in damages after fatal Autopilot crash, jury says
Published
2 days agoon
August 1, 2025By
admin
A jury in Miami has determined that Tesla should be held partly liable for a fatal 2019 Autopilot crash, and must compensate the family of the deceased and an injured survivor a portion of $329 million in damages.
Tesla’s payout is based on $129 million in compensatory damages, and $200 million in punitive damages against the company.
The jury determined Tesla should be held 33% responsible for the fatal crash. That means the automaker would be responsible for about $42.5 million in compensatory damages. In cases like these, punitive damages are typically capped at three times compensatory damages.
The plaintiffs’ attorneys told CNBC on Friday that because punitive damages were only assessed against Tesla, they expect the automaker to pay the full $200 million, bringing total payments to around $242.5 million.
Tesla said it plans to appeal the decision.
Attorneys for the plaintiffs had asked the jury to award damages based on $345 million in total damages. The trial in the Southern District of Florida started on July 14.
The suit centered around who shouldered the blame for the deadly crash in Key Largo, Florida. A Tesla owner named George McGee was driving his Model S electric sedan while using the company’s Enhanced Autopilot, a partially automated driving system.
While driving, McGee dropped his mobile phone that he was using and scrambled to pick it up. He said during the trial that he believed Enhanced Autopilot would brake if an obstacle was in the way. His Model S accelerated through an intersection at just over 60 miles per hour, hitting a nearby empty parked car and its owners, who were standing on the other side of their vehicle.
Naibel Benavides, who was 22, died on the scene from injuries sustained in the crash. Her body was discovered about 75 feet away from the point of impact. Her boyfriend, Dillon Angulo, survived but suffered multiple broken bones, a traumatic brain injury and psychological effects.
“Tesla designed Autopilot only for controlled access highways yet deliberately chose not to restrict drivers from using it elsewhere, alongside Elon Musk telling the world Autopilot drove better than humans,” Brett Schreiber, counsel for the plaintiffs, said in an e-mailed statement on Friday. “Tesla’s lies turned our roads into test tracks for their fundamentally flawed technology, putting everyday Americans like Naibel Benavides and Dillon Angulo in harm’s way.”
Following the verdict, the plaintiffs’ families hugged each other and their lawyers, and Angulo was “visibly emotional” as he embraced his mother, according to NBC.
Here is Tesla’s response to CNBC:
“Today’s verdict is wrong and only works to set back automotive safety and jeopardize Tesla’s and the entire industry’s efforts to develop and implement life-saving technology. We plan to appeal given the substantial errors of law and irregularities at trial.
Even though this jury found that the driver was overwhelmingly responsible for this tragic accident in 2019, the evidence has always shown that this driver was solely at fault because he was speeding, with his foot on the accelerator – which overrode Autopilot – as he rummaged for his dropped phone without his eyes on the road. To be clear, no car in 2019, and none today, would have prevented this crash.
This was never about Autopilot; it was a fiction concocted by plaintiffs’ lawyers blaming the car when the driver – from day one – admitted and accepted responsibility.”
The verdict comes as Musk, Tesla’s CEO, is trying to persuade investors that his company can pivot into a leader in autonomous vehicles, and that its self-driving systems are safe enough to operate fleets of robotaxis on public roads in the U.S.
Tesla shares dipped 1.8% on Friday and are now down 25% for the year, the biggest drop among tech’s megacap companies.
The verdict could set a precedent for Autopilot-related suits against Tesla. About a dozen active cases are underway focused on similar claims involving incidents where Autopilot or Tesla’s FSD— Full Self-Driving (Supervised) — had been in use just before a fatal or injurious crash.
The National Highway Traffic Safety Administration initiated a probe in 2021 into possible safety defects in Tesla’s Autopilot systems. During the course of that investigation, Tesla made changes, including a number of over-the-air software updates.
The agency then opened a second probe, which is ongoing, evaluating whether Tesla’s “recall remedy” to resolve issues with the behavior of its Autopilot, especially around stationary first responder vehicles, had been effective.
The NHTSA has also warned Tesla that its social media posts may mislead drivers into thinking its cars are capable of functioning as robotaxis, even though owners manuals say the cars require hands-on steering and a driver attentive to steering and braking at all times.
A site that tracks Tesla-involved collisions, TeslaDeaths.com, has reported at least 58 deaths resulting from incidents where Tesla drivers had Autopilot engaged just before impact.
Read the jury’s verdict below.
Technology
Crypto wobbles into August as Trump’s new tariffs trigger risk-off sentiment
Published
2 days agoon
August 1, 2025By
admin
A screen showing the price of various cryptocurrencies against the US dollar displayed at a Crypto Panda cryptocurrency store in Hong Kong, China, on Monday, Feb. 3, 2025.
Lam Yik | Bloomberg | Getty Images
The crypto market slid Friday after President Donald Trump unveiled his modified “reciprocal” tariffs on dozens of countries.
The price of bitcoin showed relative strength, hovering at the flat line while ether, XRP and Binance Coin fell 2% each. Overnight, bitcoin dropped to a low of $114,110.73.
The descent triggered a wave of long liquidations, which forces traders to sell their assets at market price to settle their debts, pushing prices lower. Bitcoin saw $172 million in liquidations across centralized exchanges in the past 24 hours, according to CoinGlass, and ether saw $210 million.
Crypto-linked stocks suffered deeper losses. Coinbase led the way, down 15% following its disappointing second-quarter earnings report. Circle fell 4%, Galaxy Digital lost 2%, and ether treasury company Bitmine Immersion was down 8%. Bitcoin proxy MicroStrategy was down by 5%.
Bitcoin falls below $115,000
The stock moves came amid a new wave of risk off sentiment after President Trump issued new tariffs ranging between 10% and 41%, triggering worries about increasing inflation and the Federal Reserve’s ability to cut interest rates. In periods of broad based derisking, crypto tends to get hit as investors pull out of the most speculative and volatile assets. Technical resilience and institutional demand for bitcoin and ether are helping support their prices.
“After running red hot in July, this is a healthy strategic cooldown. Markets aren’t reacting to a crisis, they’re responding to the lack of one,” said Ben Kurland, CEO at crypto research platform DYOR. “With no new macro catalyst on the horizon, capital is rotating out of speculative assets and into safer ground … it’s a calculated pause.”
Crypto is coming off a winning month but could soon hit the brakes amid the new macro uncertainty, and in a month usually characterized by lower trading volumes and increased volatility. Bitcoin gained 8% in July, according to Coin Metrics, while ether surged more than 49%.
Ether ETFs saw more than $5 billion in inflows in July alone (with just a single day of outflows of $1.8 million on July 2), bringing it’s total cumulative inflows to $9.64 to date. Bitcoin ETFs saw $114 million in outflows in the final trading session of July, bringing its monthly inflows to about $6 billion out of a cumulative $55 billion.
Don’t miss these cryptocurrency insights from CNBC Pro:
Trending
-
Sports3 years ago
‘Storybook stuff’: Inside the night Bryce Harper sent the Phillies to the World Series
-
Sports1 year ago
Story injured on diving stop, exits Red Sox game
-
Sports2 years ago
Game 1 of WS least-watched in recorded history
-
Sports2 years ago
MLB Rank 2023: Ranking baseball’s top 100 players
-
Sports4 years ago
Team Europe easily wins 4th straight Laver Cup
-
Sports2 years ago
Button battles heat exhaustion in NASCAR debut
-
Environment2 years ago
Japan and South Korea have a lot at stake in a free and open South China Sea
-
Environment2 years ago
Game-changing Lectric XPedition launched as affordable electric cargo bike